Motivation & Objectives
Additive manufacturing is a relatively nascent field of manufacturing that relies on the addition rather than the removal of material to construct a desired article. During the fabrication process, imperfections may occur which negatively impact the structure and surface quality of the manufactured part, among other qualities. These imperfections can be monitored and characterized while part is assembled, which allows for feedback control of the process parameters. Through an effective implementation, a higher accuracy in production can be expected, along with greater structural integrity. This will allow for greater freedom in rapid prototyping and can serve as a comparable alternative to high-precision machining. One of the open challenges is the effect of part geometry on the sensing modality and by extension, flaw detection. This is reflected in the image data recorded in-situ and can be processed to determine abnormalities as they occur.
Method of Approach
Most existing work extensively utilizes black box models or algorithms trained on specific geometries with thermal image responses. We propose to examine the time-frequency domain to generate an unsupervised monitoring algorithm to detect process faults as they occur.
- Simple Time-Frequency domain algorithm based on spectrogram implemented and used for classifications.
- Generalizations to multiple raster geometries assessed.
- Interpretable classification framework developed.
Indicative Results
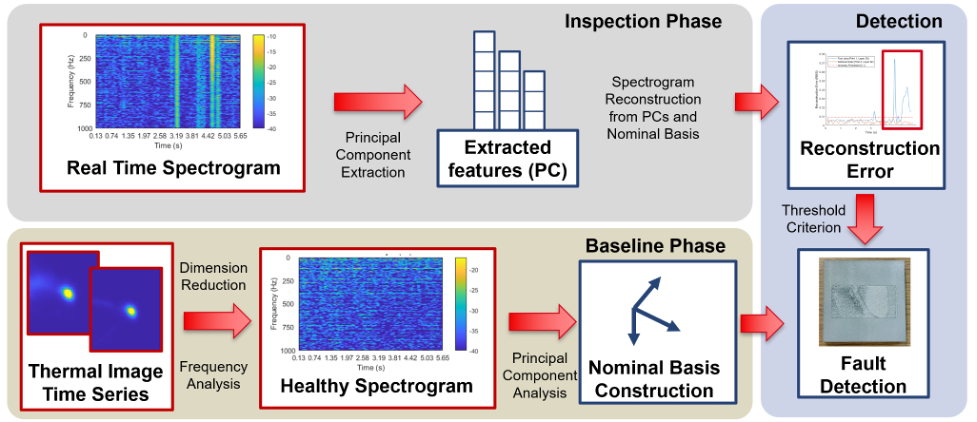
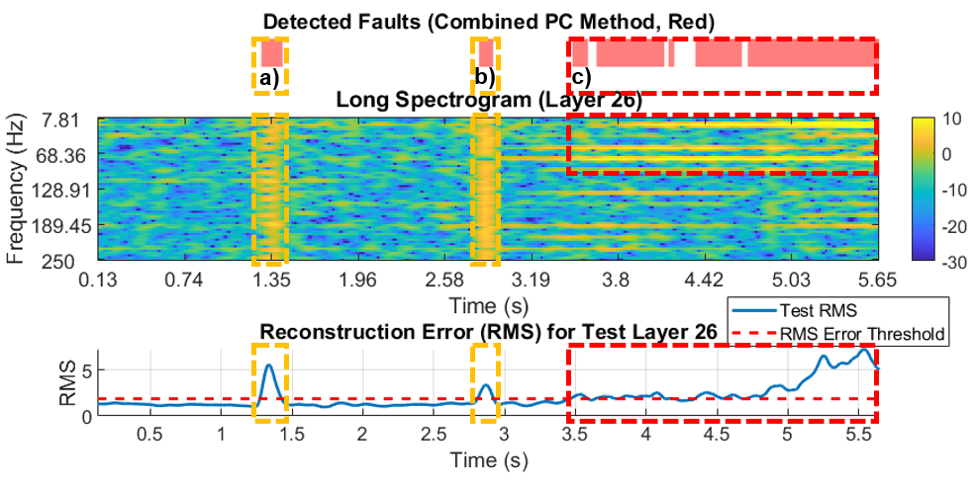