Structural Health Monitoring (SHM)
Projects
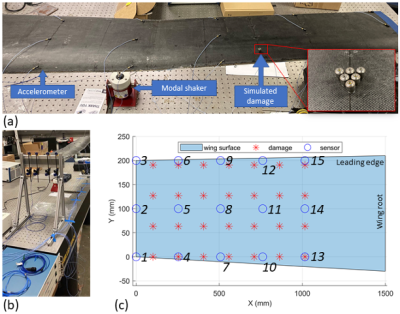
Data-driven probabilistic structural health monitoring via stochastic functional time series models
Novel sensing technologies enable aerial vehicles to continuously gather information related to their operating and structural states. Incipient damage can be detected, localized and quantified with proper implementation of onboard sensor networks and SHM algorithms. Efforts towards developing identification techniques for data-driven aeroelastic modeling, damage assessment and online parameter estimation have been previously developed. However, the treatment of constantly varying operating and structural states within complex dynamic environments remains a significant challenge that needs to be addressed.
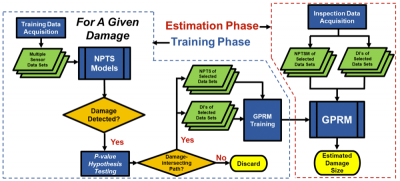
Probabilistic structural health monitoring framework based on Gaussian process regression models
When it comes to active-sensing guided wave SHM, one of the major challenges the SHM community is facing today is related to enabling accurate and robust damage detection and quantification under stochastic, spatially- and time-varying operational and environmental conditions the --oftentimes limited-- available information. In addition, the currently utilized techniques suffer from low damage/damage evolution sensitivity, need for user-defined thresholds, and non-uniform response across a sensor network, amongst others.
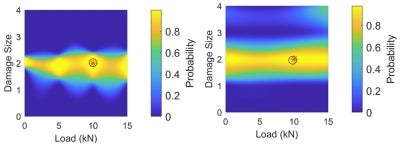
Probabilistic SHM under varying loads via the integration of data- and physics-based models
In this project, a framework was developed that promises the reduction in the training space of GPRMs along with the expansion of GPRM prediction capabilities to encompass damage size predictions under varying conditions. In addition, the proposed framework can also be used for filling the gap in the training space of multi-dimensional GPRMs as will be shown.
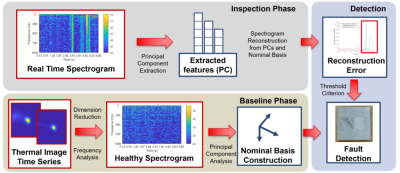
Unsupervised online anomaly detection of metal additive manufacturing processes via statistical time-frequency domain analysis
Additive manufacturing is a relatively nascent field of manufacturing that relies on the addition rather than the removal of material to construct a desired article. During the fabrication process, imperfections may occur which negatively impact the structure and surface quality of the manufactured part, among other qualities. These imperfections can be monitored and characterized while part is assembled, which allows for feedback control of the process parameters. Through an effective implementation, a higher accuracy in production can be expected, along with greater structural integrity.
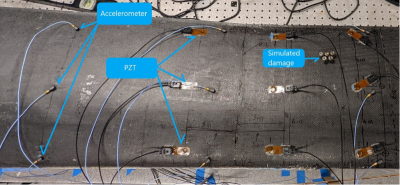
Integration of global and local structural health monitoring via multi-modal sensing
In the arena of structural health monitoring (SHM), ultrasonic guided wave-based and vibration-based damage detection and identification methods constitute two seemingly distinct approaches, oftentimes treated separately. Guided-waves-based methods are typically used for ``local'' damage diagnosis due to their increased sensitivity while vibration-based methods are based on the premise that damage has an impact on the global structural dynamic response, therefore are typically used for tackling ``global'' damage diagnosis. The challenge is that the local approach can detect small damage that does not affect the global dynamic response, therefore the global approach might miss. But local approach doesn’t provide state awareness of global dynamic performance. Moreover, as damage propagates, the robustness of the quantification can be enhanced by combining both local and global approaches. The goal of this work is to demonstrate and assess the effectiveness and robustness of the combined local-global methods both numerically and experimentally.