Students
Machine learning for reduced order and multi-fidelity modeling
Projects
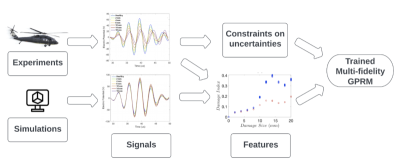
Structural state estimation via multi-fidelity Gaussian process regression models
Most existing active-sensing guided-wave methods are based on the requirement that a relatively large data set can be collected, and thus are not feasible when data collection is restricted by time or environmental conditions. Meanwhile, simulation results, though lack of accuracy compared to real world data, are easier to obtain. In this context, models that incorporate data from different sources have the potential to embrace the accuracy of experimental data and the convenience of simulated data without the necessity of large and, potentially costly, experimental data sets.
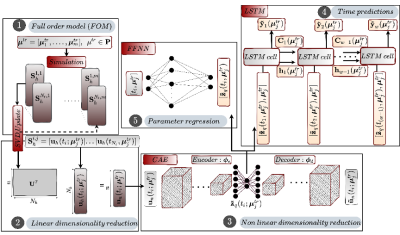
Machine-learning enabled non-intrusive reduced order modeling
The use of structural health monitoring (SHM) serves to assist the monitoring of aircraft structures thus leading to improved life-cycle management and condition-based maintenance. However, monitoring the structural behavior becomes challenging when dealing with operating and environmental uncertainties involved in both vibration-based signals and propagation of guided waves (active sensing SHM), over the variations in the material properties, loads, boundary conditions and so on. In this direction, computational structural mechanics has proven to be a unique tool in the development of numerical models that can precisely capture the operational performance of structures. Physics-based high-fidelity models (HFMs) can accurately simulate the performance of the real system and provide a useful insight while enabling enhanced diagnostic and prognostic methods. While HFMs are the backbone of the engineering design, the computational cost is increasing when simulating large scale, unsteady numerical problems. Along these lines, the demand for multi-fidelity approaches consisting of simplified techniques, able to deliver results in a limited amount of time, is growing rapidly. In this content, surrogate modeling has gained attention, aiming to reduce the computational cost of HFMs while providing results in a limited amount of time towards the realization of the digital twin (DT) concept. Reduced order modeling (ROM), as part of the surrogate modeling, has extensively been applied to decrease the simulation cost, while preserving the dominant effects of the HFM. ROMs have emerged as a useful technique to decrease the computational cost of numerical simulations and provide solutions in a limited amount of time.